5th European Forum on New Technologies - Artificial Intelligence in Chemical Engineering
13 December 2024, Paris France
Report
The 5th European Forum on New Technologies was organized in Paris on 13 December 2024, gathering experts, professionals, and researchers from academia and industry (with about a 50/50 representation academia/industry and no less than 42 companies taking part) to discuss the role of Artificial Intelligence in Chemical Engineering.
The large attendance (130 participants) coming from 17 countries confirms that AI is a hot topic that concerns many areas of chemical engineering.
The program which was structured in three sessions followed by a round-table held at the end of the day covered different aspects of AI in Chemical Engineering.
1 - Overview of artificial intelligence for process engineering
Three talks were given by Mattia Valerio, (Univ. Milano), Mathieu Cura (Optimistik) and Ludovic Montastruc (Univ. Toulouse), and here follow some of their messages to the audience:
AI may help industry to reach climate neutrality by 2050. The main obstacles to the adoption of AI in process manufacturing include challenges related to data collection and structuring, as well as the need for a near real-time data supply. However, many industrial companies have valuable data that remains underutilized and is often only accessible to data scientists. To unlock its full potential, two key steps are needed: extending data-access to the entire workforce, and applying advanced analytical tools such as AI and machine learning models.
By harnessing sensor data and using explainable AI techniques, advanced analytics are now becoming more accessible in process engineering, which is necessary to improve processes. However, conservative forces in the industry aim to maintain security instead of more openly exchanging data (internally in the company), which hinders innovation. In addition, sensor reliability remains a major problem, compounded by the slow pace of technological progress in this field over recent decades.
While academia focus on complex predictive models, the industry seeks practical AI solutions for routine issues like simulating technologies, conducting literature reviews, and optimising expenditures. In the near future, AI systems will assist operators and engineers in troubleshooting, drafting, and maintaining technical documents. Digital twins will enable real time experimentation and data analysis. Technologies will advance to create virtual environments from flow sheets, and self-diagnosing and self-optimising processes will also evolve, although widespread implementation will require time.
2 - Generative artificial intelligence and transformation in process engineering
This session included two presentations done by Artur Schweidtmann (Delft Univ. Tech) and Thibaut Neveux (EDF).
Generative AI has shown potential in tasks as language translation and automated document correction but current tool such as ChatGPT faces limitations in producing practical outputs for process engineering. To overcome these challenges, collaboration between academia and industry, increased focus on acquiring robust data, the adoption of appropriate model architectures, and the integration of domain knowledge in fields like physics and material science are needed. Hybrid models, combining physical principles with machine learning, offer a workaround by reducing data demands while improving generalization capabilities.
Generative AI excels when models integrate diverse tasks and datasets. For process engineering, multi‑agent systems could enable large language models to collaborate, interface with databases, and perform simulations. Access to high‑quality, digital engineering data is critical for advancing Generative AI applications. Universities, lacking ownership of such data, must partner with industry, as absent this prioritisation, the engineering field risks falling behind on innovation and competitiveness. EDF, a company focusing on electricity generation, employs computer-aided engineering to improve process design. They have achieved two primary goals: maximizing power cycle efficiency and shaft power, with AI generated processes closely matching expert designs and providing innovative alternatives. Future efforts aim to further automate process design and improve efficiency.
3 - Artificial intelligence applications in chemical engineering
In this session, David Robert (Technord), Anna Jaeggi (ETH Zurich), Florence Vermeire (KU Leuven), Massimiliano Villone and Maurizio De Micco (Univ. Naples) gave some examples of AI applications in different fields.
Technord uses data-driven modelling in industrial processes to enhance real-world accuracy. They combine statistical analyses, regression techniques, and traditional methods with physical mass and energy balances. They also develop software sensors to support operators and process engineers, leveraging equipment data for intelligent maintenance and pattern recognition. Their current project uses advanced process control to address disruptions and improve predictive key performance indicators for early detection and maintenance in clinker production. The company’s predictive model achieved an 84% accuracy rate after several months of monitoring and adjustment (training).
Then the characterization of crystal morphology which is a key challenge in crystallization was discussed. High performance techniques as Dual Imaging System for Crystallization Observation (DISCO) improves particle reconstruction but introduces challenges like length measurement inaccuracies due to particle orientation. Errors such as overestimating thickness or misjudging length highlight the need for refined algorithms. To improve accuracy, a test set for various particle types was created and simulations were developed to analyze shape features like circularity and convexity. A neural network reduced measurement errors by 33% compared to traditional methods. For plate-like crystals, a machine learning model tailored for precise characterization has been proposed.
The following presentation focused on property prediction models linking molecular structure to key properties especially solubility, critical for drug design and manufacturing. Key targets are solvation free energy and solid solubility, modeled using graph neural networks for solutes and solvents. A specialized architecture ensures consistent predictions for solvent mixtures. Hybrid models combine machine learning and thermodynamics, integrating quantum chemistry and experimental data to enhance accuracy. AI-powered robotic platforms optimize dye properties through iterative synthesis cycles, improving characteristics like absorption and water partitioning. These models are pivotal for optimizing molecular structures in pharmaceutical and industrial applications.
The last example dealt with the stability issues in formulated liquids, such as phase separation, creaming, and flocculation. Collaborating with Procter & Gamble, a deep learning tool using convolutional neural networks was developed for automated instability detection. Over 6,000 time-series of fabric softeners were segmented into 45,000 images, labeled as stable or unstable, resulting in 53,000 labels. Despite dataset imbalance (10% instability), advanced methods like local binary patterns and geometric augmentations improved accuracy.
An ensemble of eight models was created and validated using gradient class activation mapping, which highlighted stable areas in images for interpretability. A mosaic approach using 10-frame time lapses streamlined the labeling process. The automated detector and the high-quality "Fluid" dataset are now available on GitHub, providing valuable industrial tools for detecting and predicting liquid instability.
Round Table
The roundtable discussions tackled the critical questions:
1. Can AI enhance creativity in process engineering?
2. How can AI be better integrated into chemical engineering curricula?
3. What is the future of AI-human collaboration?
For this edition, participants were able to indicate upon registration their wish to take part in one of the round table topics which was a new feature.
Key Takeaways from the Round Table:
- The urgent need for synergistic collaboration between academia and industry to create a positive ripple effect
- The importance of democratization, within the boundaries of intellectual property rights
- Ensuring replicability of results and transparency in AI frameworks to foster trust between humans and AI
- The need to educate the next generation of chemical engineers, equipping them with a thorough understanding of AI’s potential and limitations to promote it has responsible use.
A big thank you to all participants, speakers and organizing committee (Luigi Piero di Bonito, Kevin van Geem, Daniele Marchisio, Ludovic Montastruc, Boelo Schuur).
Martine POUX
EFNT coordinator
EFCE Scientific Secretariat
Impressions
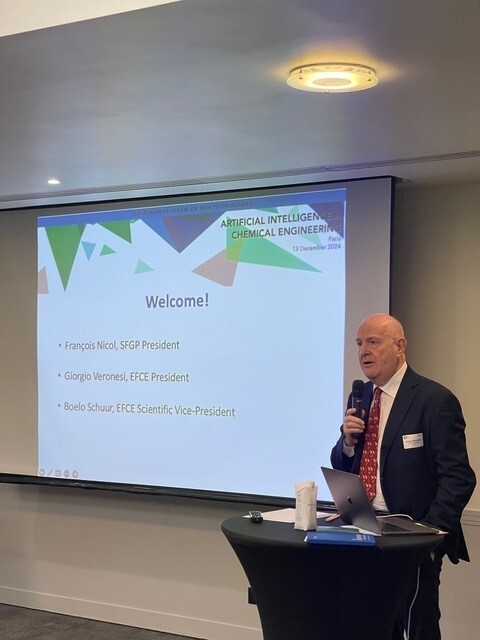
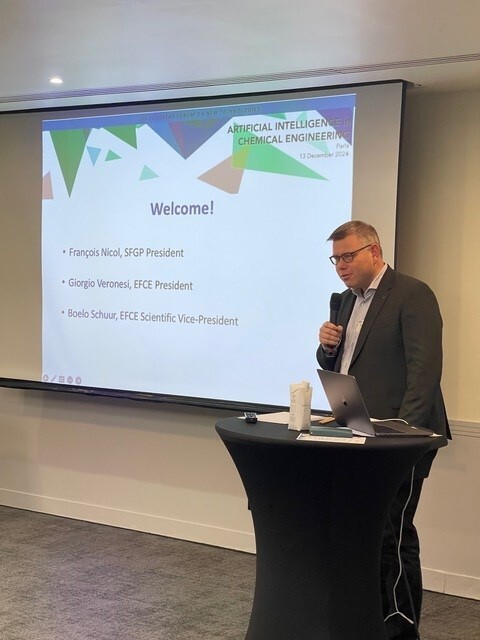
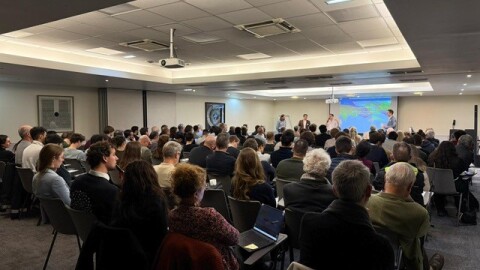
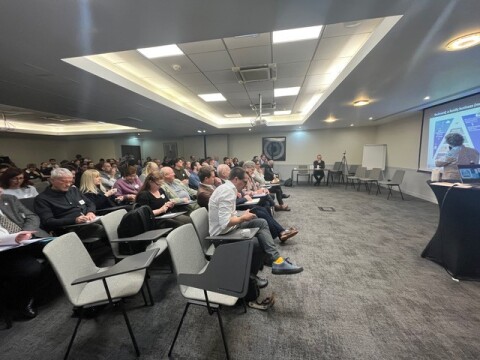
Invitation
Artificial Intelligence and Machine Learning have had in the recent past a tremendous impact on chemical engineering. On the long run they can radically change the way in which we perform research on chemical processes, we design, scale-up and optimize chemical reactors and pieces of equipment and they can potentially introduce new paradigms on chemical process control and operation.
Many are the challenges ahead for our community that can be addressed by the EFCE and its Working Parties and Sections. These can a successful journey only if the European Chemical Engineering community, both academic and industrial, is fully engaged.
In this meeting we will bring together some visionary speakers to set out the potential and the challenges. There will be the opportunity to discuss the fundamentals of artificial intelligence, deep learning and machine learning, as well as their applications to process modelling, control and the building of digital twins.
Programme
Listen & interact
Listen to the invited speakers and interact in discussions related the artificial intelligence and chemical engineering.
Recognized scientists from industry and academia will share their vision in three areas:
- Overview of artificial intelligence for process engineering
- Generative artificial intelligence and transformation in process engineering
- Artificial intelligence applications in chemical engineering
Round Table
Share your point of view on
- What are the industrialists expectations on AI?
- How could academia help develop in this direction?
- What should be taught at academia?
Flyer & detailed programme
Venue
Hôtel MERCURE Montparnasse
40 rue du Commandant Mouchotte
75014 Paris, France
Metro 4, 6, 12, 13 - Stop: Montparnasse-Bienvenue